RoboSAPIENS:
Redefining the future of robotics trustworthy adaptation
Robotic
self-adaptation project
RoboSAPIENS will develop the underlying technologies which enable robots to fully autonomously adapt their controllers and configuration settings to accommodate for unknown changes and variations while ensuring trustworthiness.
Industrial
disassembly robot
"In the remanufacturing process, used products are repaired and restored into brand-new ones of the same quality, performance and warranty, following 6 steps: disassembly, cleaning, inspection, restoration, reassembly, and testing.
The focus is on the task of disassembling – often the most time-consuming.
Manual work is still required to perform difficult disassembly sub-tasks that involve high degrees of uncertainty. To mitigate this, collaborative robots can be programmed by demonstration, but their performance is highly dependent on the type of task and the experience of the demonstrator.
It is effective for highly repetitive tasks such as pick and place, but ineffective for high-precision tasks that require force-based control to compensate for inaccuracies.
In the latter, the operator is working very closely with the robot, thus it is crucial to ensure that the re-configuration procedure is carried out safely.
With the work of RoboSAPIENS, we aim to bridge the gap of labor extensive, costly remanufacturing, and adaptable robotic collaborative automation.
A demonstration of the use case will be set up at the lab of DTI and will consist of a robot cell set up to disassemble electronic consumer waste as laptops. Complex force-based manipulations like unscrewing, un-snap fitting and destructive disassembly are needed. In some cases, the primitives will have inferior performance at the start (e.g. due to wear and tear of the different parts), thus potentially triggering an adaptation. When a task fails (due to the high uncertainty in the state of the parts to be disassembled), new primitives are constructed based on models created from human expert demonstrations.
The validation of these models includes simulated sanity checks and supervised real parts tests, as well as functional safety requirements.
If all validation checks are carried out with sufficient thoroughness, then the new skill is integrated into the robot’s repertoire.
Taking a collaborating SME laptop refurbishing company such as Tier1 A/S as a case, currently they are able to process 250k laptops per year which they foresee to increase to 1M as per the coming years.
On average a laptop takes ≈1 hour to process, where the disassembly process accounts for 0,5 hours. A Danish production worker has a salary of 28 C/hour which means they spend per year ≈3.5MC on disassembly laptops. If the process is kept manual while scaling to 1M units, the price on disassembly would be 14MC. They simply cannot scale up their business as they cannot find workforce. A solution is to send the laptops overseas, but the climate impact renders the whole process mute.
RoboSAPIENS will permit that the same number of workers can collaborate with the robots to process 4 times as many units. Saving the company 10.5MC in expenses for salary."
Warehouse
robotic swarm
"Automated Guided Vehicles (AGVs) operating on shop floors are ad-hoc machines that require specific distribution and means of transport.
The advancement towards Industry 4.0 however, calls for the use of Autonomous Mobile Robots (AMRs), a more versatile and affordable option than AGVs, consisting of robots equipped with a mobile base and even robotic arms, allowing them to autonomously navigate and perform dexterous tasks without any additional physical equipment.
It is envisioned that these robots will be deployed as a fleet on the shop floor, able to navigate freely and safely, while taking into account changes in the fleet and the surroundings (e.g. the number of robots, blockages by humans, waiting for robots), corresponding to self and environmental awareness respectively. When such changes incur, RoboSAPIENS will provide a solution to dynamically adapt the work assigned to each member of the fleet and the navigation through paths.
Such adaptation will take dynamic parameters into account, such as disconnected robots, battery status, proximity to goals, past human behavior, etc.
The case study comprises a fleet of robots of PAL Robotics’ in the TIAGo family. The scenario consists of these robots set in the shop floor, controlled via a fleet management system.
During their operation, one or more robots may come and go (e.g. due to low battery), communication between robots and the fleet management may drop, emergency exits may be blocked due to stopped/malfunctioning robots cutting supply chains and endangering humans, or the floor plan itself may change.
Such anomalies will trigger the monitor, and the state of the fleet and the environment are re-evaluated. The TIAGo robots are capable of Simultaneous Localization and Mapping (SLAM), and their sensor readings are used to update the map and inform the fleet manager. The planning phase is carried out by adopting a genetic algorithm to reschedule tasks. After the system is validated through simulation, and the self-adaptation process is deemed trustworthy, the model is deployed to the fleet manager.
The current algorithm replans and re-optimizes the robotic fleet but does not consider the fact that new warehouse floor plans and humans touring may cause robots to obstruct crucial pathways such as an emergency exit, become a potential safety hazard, nor does it account for new robots entering/leaving the fleet.
Through RoboSAPIENS it is expected that these optimization algorithms solve the problems but with an added set of constraints that ensure the trustworthiness of changing shop floor plans as well as the number of robots.
A change in the floor layout currently requires a remapping effort that comprises: stopping entire fleet, identifying changes to physical layout, replanning, and restarting the fleet. It is thus a tedious process that takes hours.
After RoboSAPIENS, it is expected to be done online, hence in seconds. If a robot stops working, or a group of touring humans block a passage, they will be immediately considered a blockade, and the fleet manager will replan accordingly. The fleet manager and robots will closely cooperate into comparing their environment with the expectations."
Human-robotic
interaction
Risk assessment is a prominent concern in human- robot interaction for cobots.
It is an iterative process that systematically identifies hazards and specifies measures to reduce these hazards’ probability.
The procedure and requirements are specified in the Machinery Directive 2006/42/EC and harmonized safety standards. The current manually operated and strongly heuristic practice stands in bizarre contrast to the paradigm of Industry 4.0.
Ignoring data during the risk assessment leads to a loss of efficiency in safety engineering. This is particularly evident in production systems featuring human-robot collaboration, where people and machines work closely together. In this case study, we aim to use system and sensor data in a dynamic human-robot safety model to automatically assess the risk, to improve the overall production system’s efficiency and to significantly reduce the costs associated with risk assessment. This way, the risk assessment of collaborative machines is subject to self-adaptivity, thereby keeping or even augmenting the safety of the humans in such environment.
A demonstrator of the use case will be set up at the lab of Fraunhofer and will consist of a collaborating robot and vision-based sensor technology in a defined workspace. The robot has to be equipped with the necessary safety functions to allow direct collaboration as a form of interaction.
The sensor technology has to monitor the position, movements and states of the human in combination with Deep Learning. The application scenario includes an industrial assembly task that is representative of typical
With these data, the original assumptions on the human movements can be reviewed. If a deviation from the original assumptions is detected by monitoring unanticipated human and machine behavior, the risk model must be updated and assessed. With risk management as part of the Digital Twin, it can then be determined whether collision avoidance or management is permissible. Thereafter the residual hazard is evaluated, and the measures are validated.
If the self-adaptation process is deemed trustworthy, the system implements the identified countermeasures.
Human safety is a crucial factor for hybrid production systems in which people and machines work closely together. Static assumptions can describe the high-hazard dynamics in such systems conservatively at best, which commonly degrades their performance unnecessarily.
Data and models of such human behavior will therefore be subject to self-adaptivity, thereby tackling the challenge of risk assessment in both the observations and the models. Safety validation will be a prominent goal in this case study.
Currently, collaborative robots can employ models of the humans nearby to track them and thus prevent collisions. These models are however static and, if the layout of the factory changes, need to be reprogrammed, an activity that takes days.
Through RoboSAPIENS’ digital risk assessment and the concept of dynamic risk estimation, significant reductions in safety engineering costs of up to 80% and productivity gains of up to 10% are possible.
Prolonged hull of an
autonomous vessel
"Estimating the motion of the ship in the immediate future, either from a dynamic model, or a data-driven one using adequate historical data, could support autopilots and thus improve the safety of autonomous ships.
However, deploying the prediction system to new ships without enough prior knowledge of their dynamic behavior deteriorates navigation capability, especially in the presence of environmental uncertainties including wind, currents and waves.
Identifying model parameters via sea trials or collecting the needed data for ship motion modelling will take a relatively long time. In this case-study, we therefore leverage the dynamic model from a reference ship and the limited available data from the target ship to build up a transferrable model that can represent the target ship motion.
The plan is to take the Gunnerus research vessel as a case study of the self-adaptation of a representative model for motion prediction to ensure safe ship motion prediction. Gunnerus has been prolonged by 5m in length in 2018.
While there is a high-precision dynamic model of the original vessel, it cannot directly be used for the prolonged vessel, for which we have limited sea trial data. In such a context, we aim to apply Deep Learning to the prolonged vessel, by combining the old dynamic model with limited real motion data to generate a ship predictor. In the case study, a motion calibrator will be first created based on the motion discrepancy from the model-based predictor and real data, and then incorporated into that predictor for motion prediction.
When the ship’s motion predictor underperforms a monitor is triggered. Data is recorded from the trigger time to a predefined later time and used to update the transferred prediction model, which is trained using Deep Learning.
If the validation passes and it is deemed trustworthy, the updated model is deployed and thus executed, otherwise the system goes back to the analyze phase. Additionally, investigations about what is a suitable amount of data needed for the transferable model, the impact on the prediction performance, and the generalization of the transfer modelling will be conducted.
Accurate ship motion predictions play a vital role in providing onboard support for decision-making. However, some cases in real life such as when the load of a ship is changed, or a ship is reused after a retrofit, have usually neither a precise ship model available, nor enough data that can be used for training the ship motion predictor.
As a result, it takes relatively long time to collect data, e.g., from a sea trial, towards either mathematical modelling or data-driven modelling for ship motion prediction. This is not an economic solution. Instead, a predictor making use of a rough model and updating online employing recent historical data collected during navigation is preferable for the maritime industry.
In such a context, the main challenges lie in the inaccurate ship model, limited training data, and environmental disturbances. The main concern of this use case is how to adapt the ship model and apply it for ship motion prediction during navigation.Before RoboSAPIENS the predictor of an autonomous ship has to be updated based on recent historical data at fixed time intervals. This is because in order to adapt to environmental changes, the predictor has to be refreshed regularly, even when the environmental effects are small. This will increase the update frequency, the workload for commissioning, and the interaction with captain and onboard crew for approvals. Current cost estimate: 20,000 to 50,000C/year.
After completion of RoboSAPIENS we will provide tools to force the predictor for update in a safe manner, the trigger of update of the predictor will be dynamic. Taking advantage of the safety layer, we can cut down the cost for commissioning, reduce the interaction frequency with captain to some extent, while maintaining prediction accuracy within an acceptable range. The updated cost estimate is less than 10,000C/year."Human-robotic interaction."
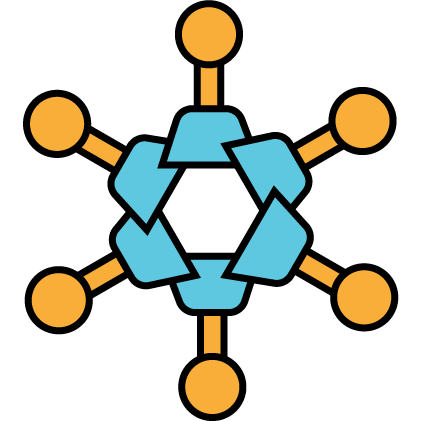
Join the RFG
Are you interested in becoming a member to unlock collaboration and knowledge-sharing within our industry network?
Subscribe to our early bird list now and you will be notified once the Group is ready!